Saturday Links: AlphaFold3, The SEO Storm and Mapping LLM Concepts
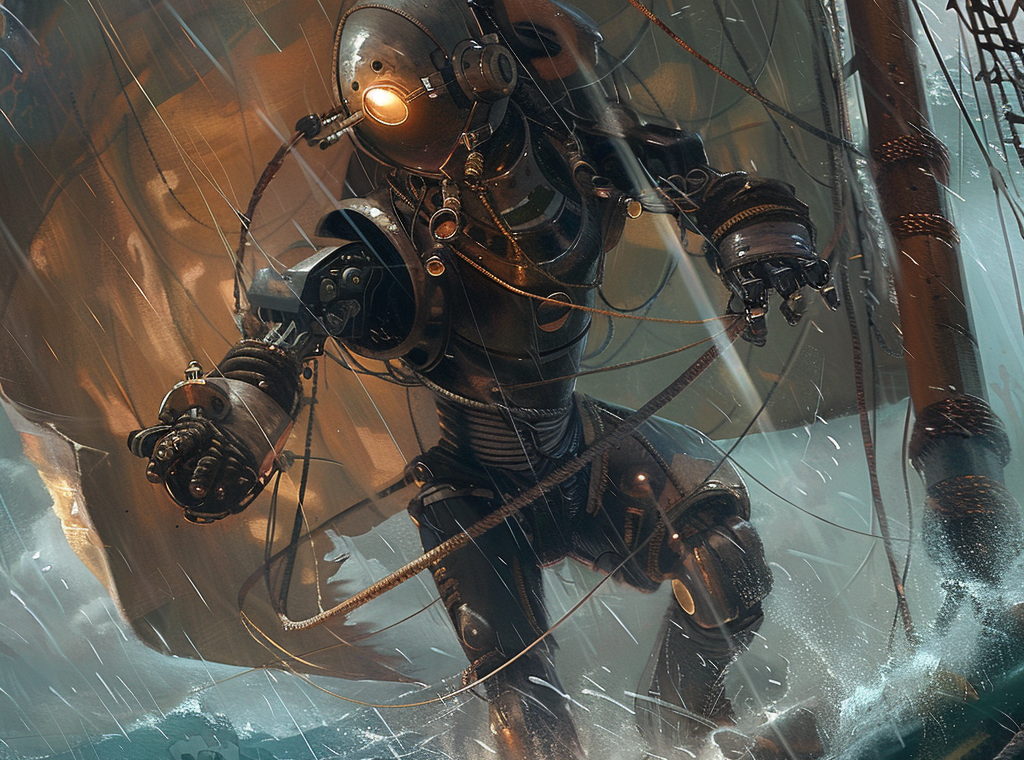
Here are this week's links:
- AlphaFold 3 now predicts how proteins interact. Google's AlphaFold project is already one of the best examples of using AI to understand the biological world. Versions 1 and 2 were able to predict possible protein folding configurations, which was a major breakthrough. AlphaFold 3 takes these capabilities even further and can often also project how molecules will interact with each other. This represents another huge leap forward in our understanding of biological systems. Unfortunately, the newer variant will no longer be open source. Though there will be some free access, I guess it's clear that at some point, Google will want to recoup its investment here.
- Google just updated its algorithm. The Internet will never be the same. The title is a little clickbaity... but this is a decent unpacking of the roiling challenges in Google SEO at the moment. Both Google is trying to ensure its search results are relevant (and not just gamed pages that somehow trigger its algorithm) and the sites being ranked to try to rank higher. The looming change everyone is anticipating is a shift to "AI Answers" in search results, which will likely mean many people don't click on the (lower down) search results at all. There will be links in those AI answers (so it'll be advantageous to be there), but far fewer than in the long list. Small publishers are likely to see significant drops in traffic (that seems inevitable), and there will be rage at Google for the changes. However, it's not clear why Google really has a choice: AI answers will be expected, and users will flock to Meta.AI and ChatGPT instead if Google doesn't provide inline AI. The real question is, how do we find new ways to facilitate discovery for small and midsized valuable content? The general technology trend when the distribution is nearly free tends to be that there are only two ways to win: 1) being a giant aggregator and 2) being a tiny micro-player with a dedicated fan base that is willing to pay.
- Another content deal for OpenAI. As regular readers will know, one of my regular topics: content deals for training and usage. The deal gives OpenAI access to NewsCorp's archive and (if it's similar to the Axel Springer deal) likely to also surface news content (and links) in responses. The former is presumably for training, the later is presumably to appease news organizations and give them back traffic from results. The deals raise tons of questions: are OpenAI suggesting that they need to pay for training data? (They likely will argue no, it's just convenience). Will this force other model makers to pay, and are they raising the cost bar for others deliberately? Once you have content from a few big players, do you need anyone else? What does ChatGPT prefer? The Daily Telegraph, the New York Post, or the Sun Newspaper?
- Anthropic's conceptual mapping helps explain why LLMs behave the way they do. A nice summary of Anthropic's research at ARS Technica. The work shows the way clusters of neurons are associated with concepts during inference in an LLM. It also goes further into what happens if certain concepts are pushed to high levels of activation. It's early work, but one thing is clear: LLMs are a delicate balance of interwoven concepts. While this looks tantalizingly like we could "amend" behavior by interfering at the concept and neuron-level, I suspect it means exactly the opposite: almost any change could have complex waves of unintended consequences.
- ‘Don’t focus on LLMs.’ In a topic he's returned to regularly, Yann LeCun underscores that we need to go beyond LLMs for realistic reasoning. He also made the point that focusing the efforts of individuals or small companies on LLMs themselves probably doesn't make sense; it's likely to be a large company game. I think he's right on the former. LLMs will ultimately be a component of larger neuro-systems. On the latter... from a rational perspective, he's probably also right. Given that Meta is willing to spend 100's Millions to make very capable models and open-source them, it's hard even for well-funded companies to compete unless they are one of the 4-5 top players. On the other hand, we barely understand how LLMs work still. There are (I believe) tons of breakthroughs waiting in how to improve them, how to minimize their size etc. For those problems, small team constraints might be good!
Wishing you all a wonderful weekend (extended into Memorial Day if you are in the United States)!