Sunday Links: AI Trillions, Ontologies and Weather Balloons
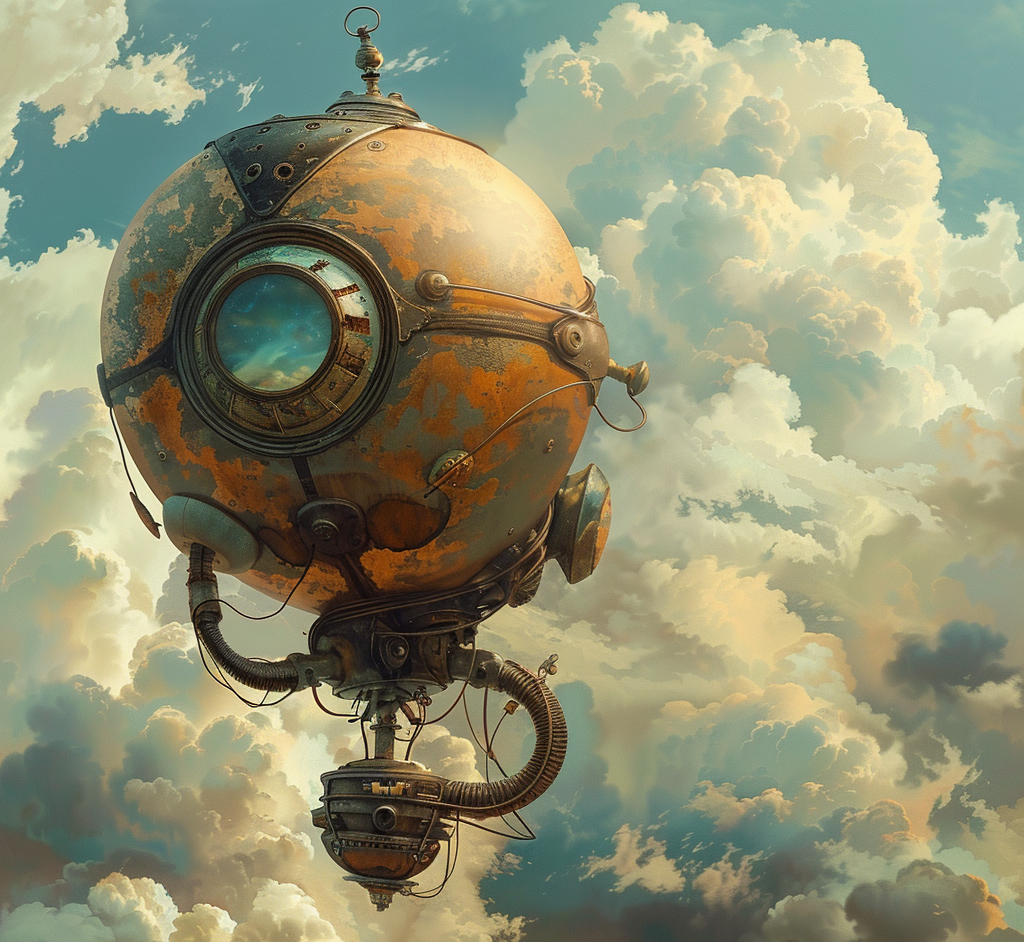
A little late again this week after a lot of travel. Here are the most interesting links I came across:
- NVIDIA's Market cap. In other news, NVIDIA's market cap rose to over $3Trillion this week, briefly overtaking Apple. NVIDIA crossed the $1T mark in May 2023 and the $2T mark in February of this year (4 months ago). Luckily, Jensen's Hwangs, now iconic leather jackets, have survived all the transitions so far. Perhaps rivals need to figure out how to shut down his supply!
- AI Antitrust. At the same time, and perhaps not entirely unrelated, the US DoJ and FTC have announced an agreement to look at potential anti-competitive practices by big tech players in AI (including NVIDIA, OpenAI, and Microsoft). This seems very premature to me. The AI market has only existed for 18 months, and a company like NVIDIA, in particular, has arguably also been a huge factor in unlocking the market's potential. It's possible there are some exclusionary practices in play, but it seems very clear that NVIDIA's gains, in particular, come from a superior product. There is plenty of activity to try to eat their market share, but it is all falling short thus far. If, in 5 years, we still only have one primary supplier, this case may have more teeth. As for Microsoft, they may be in hot water for their unusual deal with inflection. None of this means we should not be vigilant, but antitrust should be about the long-term abuse of a monopoly, not the potential for abuse due to a business advantage.
- Cube is building a ‘semantic layer’ for company data. This is not a new concept: there are multiple companies working to develop semantic graphs and ontologies for data sets in the AI era (Timber.AI and AtScale are examples for DataBricks). The basic concepts go back to Doug Lenat's Cyc project and to category theory beyond this. By creating a graph of relationships between concepts we can better put any single piece of knowledge into context. One can argue that LLMs learn these relationships implicitly from their training data. However, adding explicit ontologies and also linking them to data sets of smaller AI systems that are not ful powered LLMs will increasingly be useful to have shared knowledge context. I'm not sure to what extent these concept map systems will be bought from individual companies such as Cube, arise in the commons, or be part of more general AI offerings. In any case, this "semantic" glue will likely be something enterprise AI will benefit from especially. The Wikipedia article on Ontology is (perhaps unsurprisingly) a very complete first primer on ontologies.
- Pure generative, meet procedural. The link is for a (newish) add-on for the 3D modeling tool blender, which uses procedural rules to create buildings, city blocks, roads, etc. The point of adding it here isn't to say that this plugin is a huge breakthrough in and of itself. Instead, it sparked a point that we're often missing in Generative AI discussion. LLMs, diffusion models, etc., often generate content with a large amount of unexpected flair and creativity. Each creation is picked out of the ether of random numbers. On the other end of the spectrum, we've gotten better and better at procedural generation. This is a set of techniques that take collections of rules and parameters and create complex, intricate models of almost anything. Run at small scales; everything looks unique; at large scale, often some repetition kicks in because the rule set only contains so much. The strength of procedural generation, though, is that it is generally grounded in some realistic rule set, so all outcomes have some real-world logic to them. It seems to me that the big win will be to marry the two approaches (genAI and procedural generation); that way, you have infinite diversity but a grounded process for checking validity.
- WindBorne Raises $15 Million to Scale Its Balloon Constellation and Bring AI Weather Modeling to the Fight Against Climate Change. Windborne operates a network of weather balloons that pack enough sensors to see more than standard weather data gathering stations (and satellite data). The company then fuses this data with other data sources and is producing an LLM-based advanced weather model. It's great to see the buildout of new data sources (this may also give them some defensibility) and also to see advances in something (weather prediction) where it's seemed for many years we were at the edge of what was possible.
Wishing you a wonderful Sunday!