Sunday Links: Solving Math Problems, Materials and Integrating World Models with LLMs
AI for Science can be truly transformative
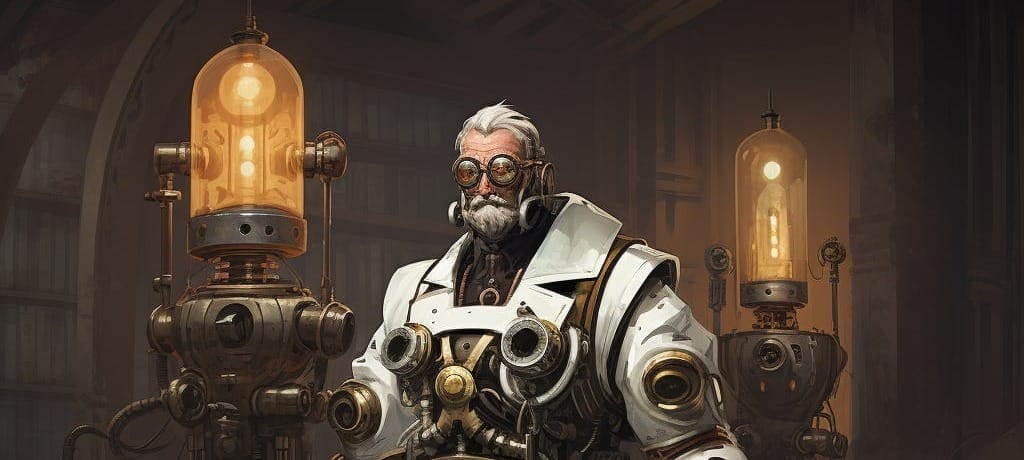
These should have arrived on Friday, but the week got away from me, and I couldn’t complete. Regular service will hopefully be resumed next week!
This week, I decided to focus on areas of AI and LLMs that are emerging and perhaps under-appreciated in the general discourse: breaking new boundaries in scientific & engineering discoveries.
When one asks the question: where can AI bring amazing value? One of the answers is giving superpowers to people already on the frontier of humanities knowledge on something. The ability to predict and suggest things to try (or evaluate them fast) can be an incredible boost. Even more important, a single discovery could be tremendously valuable.
This week’s links in this category:
- Google Deepmind’s Codey LLM is used to crack unsolved algorithmic problems. This gets at the heart of AI for science. We’re used to LLM’s “hallucinating” and thinking it’s a bad thing for serious applications. However, by coupling specialized LLM and a scoring system, the deepmind team has shown you can find solutions to problems that humans haven’t uncovered yet. The problem solved is the Cap Set problem, which involves calculating the largest possible set of something against a constraint. While this isn’t earth-shattering itself (though it is a famous problem), having a system that can produce answers to such things is important. The system is not just producing code to solve a known issue, it’s inventing novel algorithms.
- AtomicAI focuses on LLMs for predicting RNA-based therapy outcomes. RNA and gene therapies, in general, are another area where there are explosive numbers of combinations to try. It’s hard to predict what the algorithms might find, but the thing they do that works might have a tremendous impact.
- Google launches MedML Generative AI for Medical Applications. Having medicine-specific generative AI seems to be a clear avenue for breakthroughs. While many applications are likely to be in treatment, some mentioned are in research (like identifying correlations between diseases and biomarkers). As a good friend of mine pointed out, the team behind MedML is not very visible at Google; perhaps for something so sensitive, it would be good to have more information revealed on what’s going into the system.
- We already covered the potential for AI-discovered materials in a previous Links post. There was a workshop focused on just this topic at last week’s NeuroIPs conference and all the accepted submissions are published and linked on the workshop website. So for a true deep dive on the cutting edge, this is a great place to look.
- General World Models initiative from Runway ML. Coming at this “search” problem from the other end (decidedly unscientific) is Runway MLs R&D effort to infuse video generation with basic world models. This is one of the obvious evolutions of how LLMs are used. We’re far away from being able to do “science” this way, but ultimately it’s the same problem: using LLMs to do ideation and and search in a constrained world model. Dog-level AIs are the new frontier.
The defining feature of “when this works” is that the problems are “trapdoor functions”. The trapdoors (to a great solution) are hard to find, but once you fall through it, you know that you did. It needs to be relatively easy to verify if a solution is good or interesting and with many possibilities to try. If you get even a little better at guessing which combinations to try it can be a big advantage.
In somewhat related news, some researchers at UCL may have a start on a new unified theory of physics.
A new study has also flagged that the number of highly productive (by volume of papers) researchers has risen sharply in some fields and countries. Factors like tenure rules and academic status may be driving these spikes for now. An interesting question is whether AI will be another factor and how much of a good or bad thing that is going to be. Hopefully, the absolute best Human+AI papers will have such breakthroughs, and the likely increase in mediocre papers will be a reasonable price to pay.
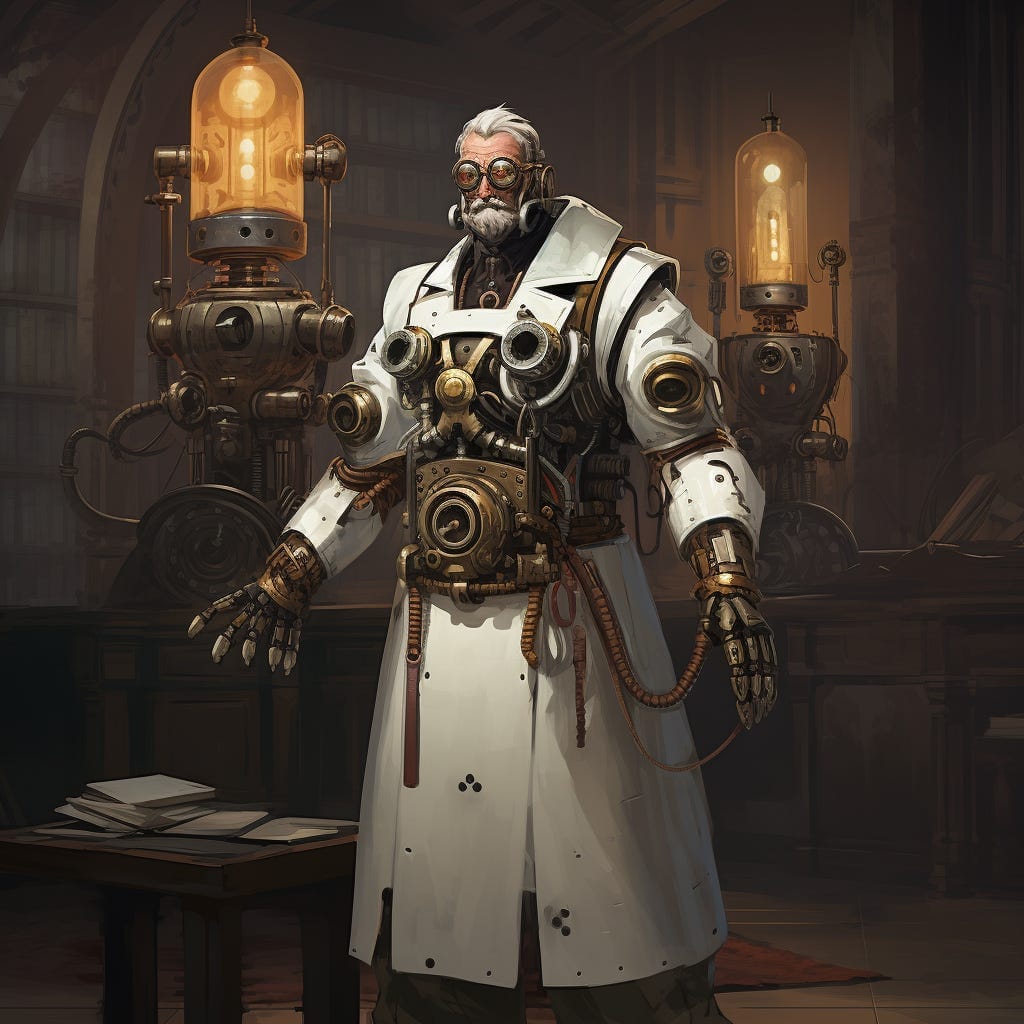