Your AI Startup's Data Moat Might Not be as Deep as it Looks
New techniques are getting more out of less data and not all data is created equal
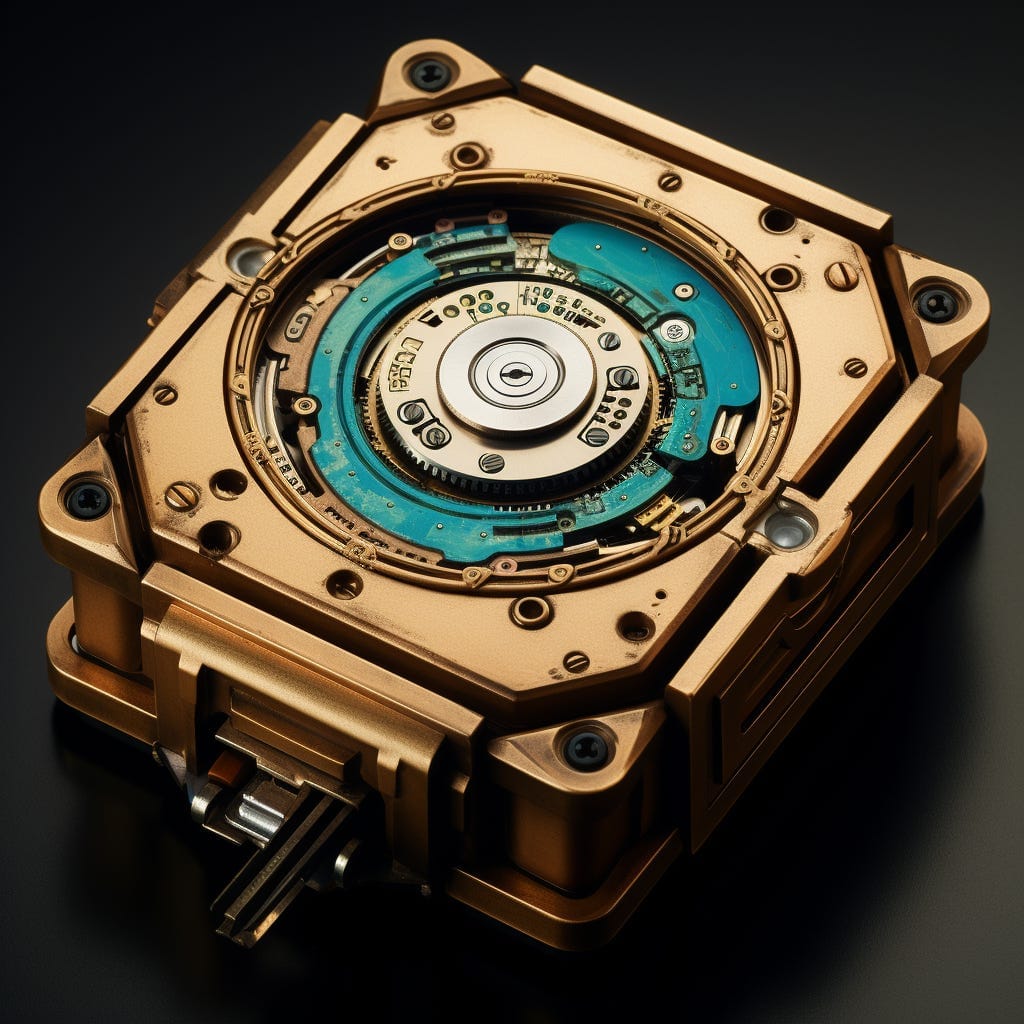
One day late this week due to some international travel!
For many of the AI companies launching over the past twelve months, one of the key arguments for a quick launch (and for investors to invest early) has been the plan to build a data advantage: building up a body of training data that gives them a lead over competitors. More data leads to a better-trained AI model, which leads to happier customers and even more data.
This works in certain situations, but in others, the argument is a lot thinner than it may have looked a few months ago. There are a few reasons for this:
- First: more data is not necessarily better. In some applications, early may look for different things to the larger market and continuously try only a tiny range of queries. This means there is a premium on reaching specific market niches to get data coverage and a need to try to nurse people over the initial “newness” of AI. A cost that later entrants might not have to pay. Speed still helps, but unless efforts are made to try to reach a broad range of potential use cases, the head start might not lead to a sufficiently rich data set.
- Second: it’s become more evident that in many B2B applications, there will be a considerable reliance on customer internal data for training per customer rather than the smarts of the actual AI model. (e.g., in RAG style setups that will likely be dominant in many fields, including legal, regulatory, support, and others.). In these cases, the most potent leverage is the ability to quickly and accurately train with and use a new data set, not locking in extensive data sets early.
- Third: new techniques such as RLAIF (Reinforcement Learning from AI Feedback) are starting to show the potential of squeezing more out of a single training data set. Specifically, the technique replaces the feedback often derived from human evaluators (often customers) with pre-coded evaluation functions. The system then generates new sets of answers that are filtered and used for training again. There are dangers to these techniques, but it seems clear that there will be at least some automatable gains. This type of procedure will likely be most effective for domains where a bounded number of queries make up the bulk of customer requests (e.g., customer support chatbots). In domains with many specific outliers, larger data sets will still be more likely to win out.
- Fourth: Training is also speeding up quickly (see techniques such as Würstchen for images, for example, though advances for almost every model type are coming thick and fast). These speedups mean that CPU time and money spent today crunching a large data set might be twice what would be paid in 3months.
None of this is to say it’s not good to start early or get access to data more quickly than others if you’re building in a space, you absolutely want to get started as fast as you can.
What it does mean, though, is that you might want to slow down spending on early customer (but especially data) acquisition to make sure each new dataset is giving genuine differentiating value. Further, don’t rely on your data as an effective moat unless it’s literally secret and valuable. Other players in the market who focus on tools first and get good at training or onboarding data before entering the market first might fly past, no matter how much of an early data lead you built.
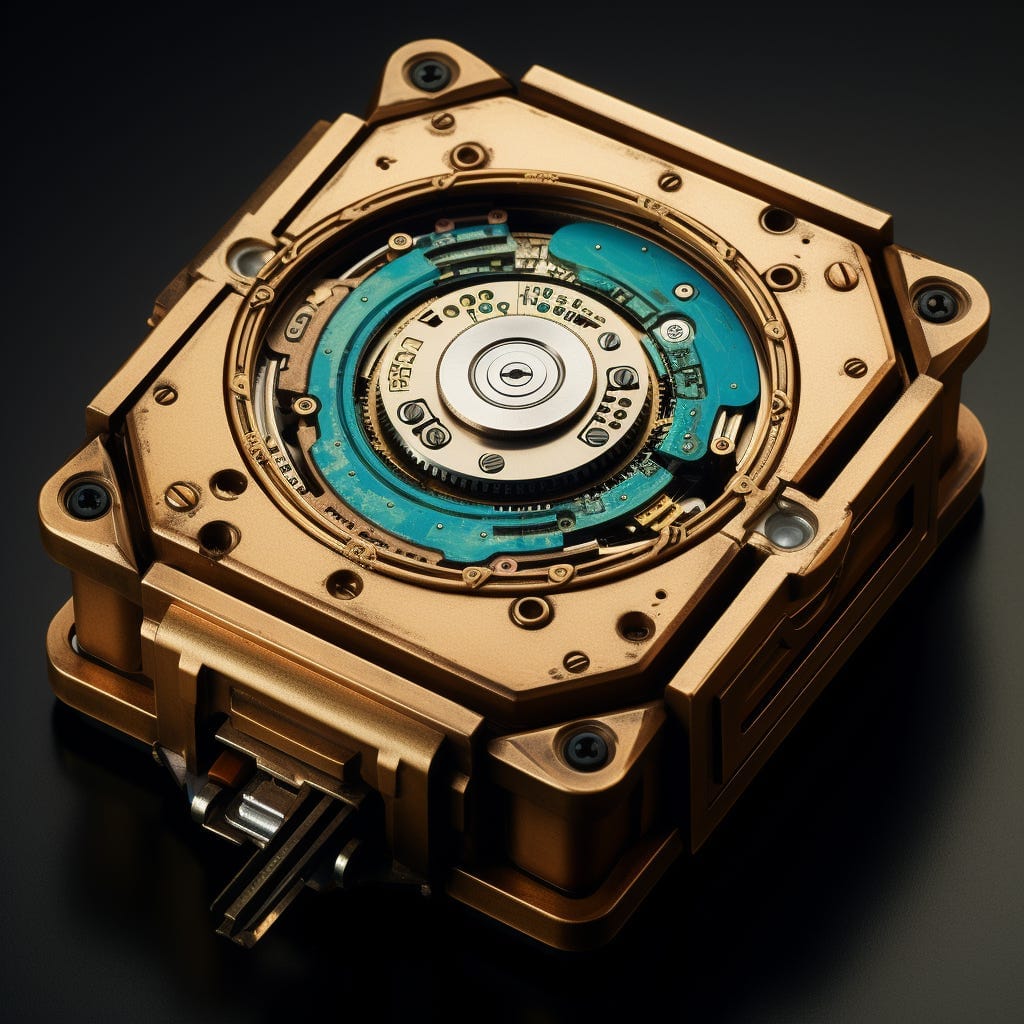
What’s the Value of your Data?
The training data needed to produce a good ML model typically takes the form Query : Answer : Score. The query determines what question is being asked. The answer is a response, and the score indicates the quality of the response. (The latter might always be implicitly 1 if all answers are assumed to be good.).
There is certainly value in getting a significant volume of possible questions and answers. One of the critical values of the Alexa smart speaker to Amazon over the years has undoubtedly been the many 100s of millions of queries and responses it has recorded.
This data gathering also highlights some key problems, though: how many were really unique? (It’s hard to imagine that more than 50% weren’t just queries for the time, the weather or set a timer!). In Amazon’s case, these were likely still very valuable since they came in a myriad of different accents and sound quality situations. For the challenge of human voice understanding, the more, the better. Unfortunately, in other applications, this type of heavy concentration might mean much of the data is low value. (I wonder if Inflection.AI might suffer this fate early on since it’s a text-based system with a very generic remit.)
Not all data confers the same value:
- General interaction information: user questions and answers that are relatively general and would be answered similarly in many contexts (e.g. password resets, account name changes, refund requests, etc.). Getting specific questions from one set of customers is unlikely to provide differentiated insights that another company might get from a different customer set.
- Restricted licensed information: this includes textbooks, movies, artwork, and anything else copyrightable. Securing the rights to train on such data could mean a significant advantage. This is particularly true if a company is the sole data owner and can hence decide not to license to anyone else. In many cases, this could put incumbents in the driving seat, but in the current early market, there may well still be access to assets that are undervalued, and licenses could be locked in for a lengthy period.
- Unique proprietary and secret information: situations where a company has its own records of not only proprietary answers to questions but of records of the questions asked are not public. This may be the case with a law firm and all its case notes or consultancy and its customer deliverables.
- Real-time information: access to a real-time data stream that may change rapidly and require model adjustment. Examples here include fraud detection, cyber security, or reacting to breaking news. In this case the larger the data flow, the more likely it is essential signals will be in the sample. (The infrastructure to adjust model behavior at a speed like this is itself highly specialized, with players like Tecton providing infrastructure.)
It’s helpful to ask which types of data are needed for the application at hand and how much it can be secured for use by one company versus being available to others or even being generally available.
High Leverage Activities
Given that competitive moats don’t automatically emerge from a data advantage, what can you do to shore up a competitive edge? A few good tactics here are:
- Try to ensure customer usage of the system produces artifacts that enrich the system itself: A great example of this is Stability AI’s Stable diffusion, in which many of the users actively fine-tune the model and share those fine tunes. Leonardo.AI and Scenario.GG also encourages users to share their custom models. (*). In applications like these, user engagement goes beyond simply using the system and generating input for new training but invests users in the actual training itself and the sharing of those results.
- Get really good at ingesting customer proprietary data to create a tuned model for each customer: in domains such as legal or support bots where the data separates into a somewhat generic interaction layer and a deeper layer of specific data unique to each customer, focus on getting good at data onboarding. Having early examples helps here, but the number of customers likely isn’t that important. What is more important is being able to scale customer onboarding fast as demand ramps up.
- Build a domain map: any AI model you build is based on the training sets ingested, so having an understanding of the distribution of possible data in the domain is critical. The more you can map out what is possible, the better. There is still debate as to how valuable synthetic data is in filling in gaps, but techniques like RLAIF as mentioned above, suggest that this may well be valuable. With some judicious engineering and data creation you may be able to stretch what you have further.
- Get access to unique, useful data sets: this one is obvious, but in those domains where there truly is individual data, it’s extremely valuable to access the scarce resources available. These may be via licensing or a third-party company that is generating this data as a by-product. In some cases, by creating the data yourself (though if you can do it, others might also be able to).
Investors will be increasingly less convinced about data forming a moat for AI startups, so any of these you can pull off will help in an investment discussion.
Conclusions
The AI boom runs on data, and getting data early could drive significant advantage, but it’s often less clearcut than it looks. Establishing a moat with an AI application is still very similar to winning with any other type of startup: capturing customers and retaining them is ultimately more important. Much of what keeps customers returning might be in how you ingest and adapt to new data rather than the strength of the model trained on the first data sets you encounter.
Have a great week!
Notes
- (*) For full disclosure, Piton Capital is an investor in Leonardo.AI.