Can AI really be Creative?
Yes, unless you want to discount most of human creativity
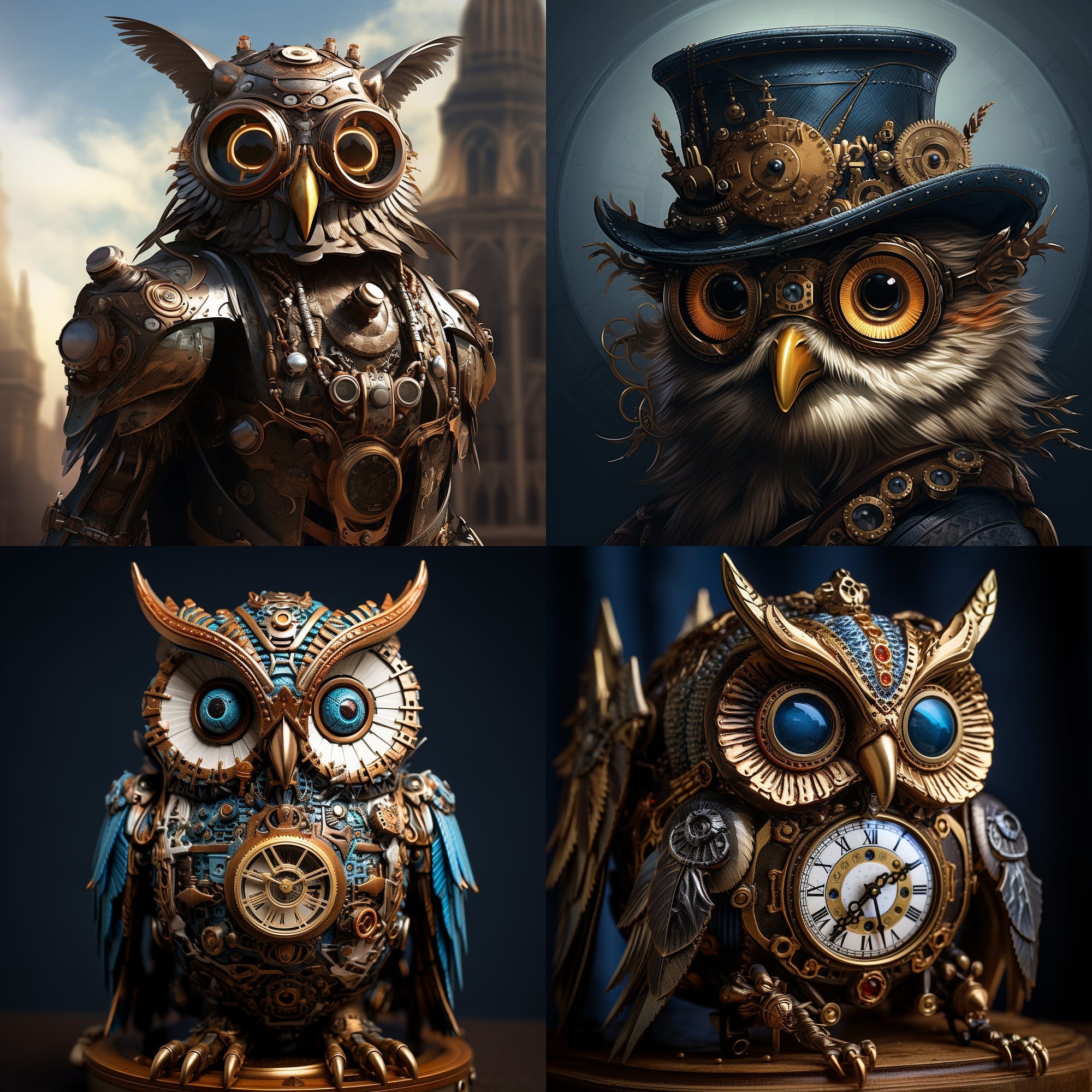
This is a long post. So the TL;DR is: no matter how you define creativity, it’s tough to see how AI doesn’t meet the definition. That may “ding” our sense of exceptionalism as humans, but on the bright side we’ll see AI (and especially humans + AI) create some exceptional ideas and art. From an investor perspective, if you see pitches that say “We’re building AI to invent new stuff in domain XYZ”, then it may be a lot less of an oxymoron than it sounds.
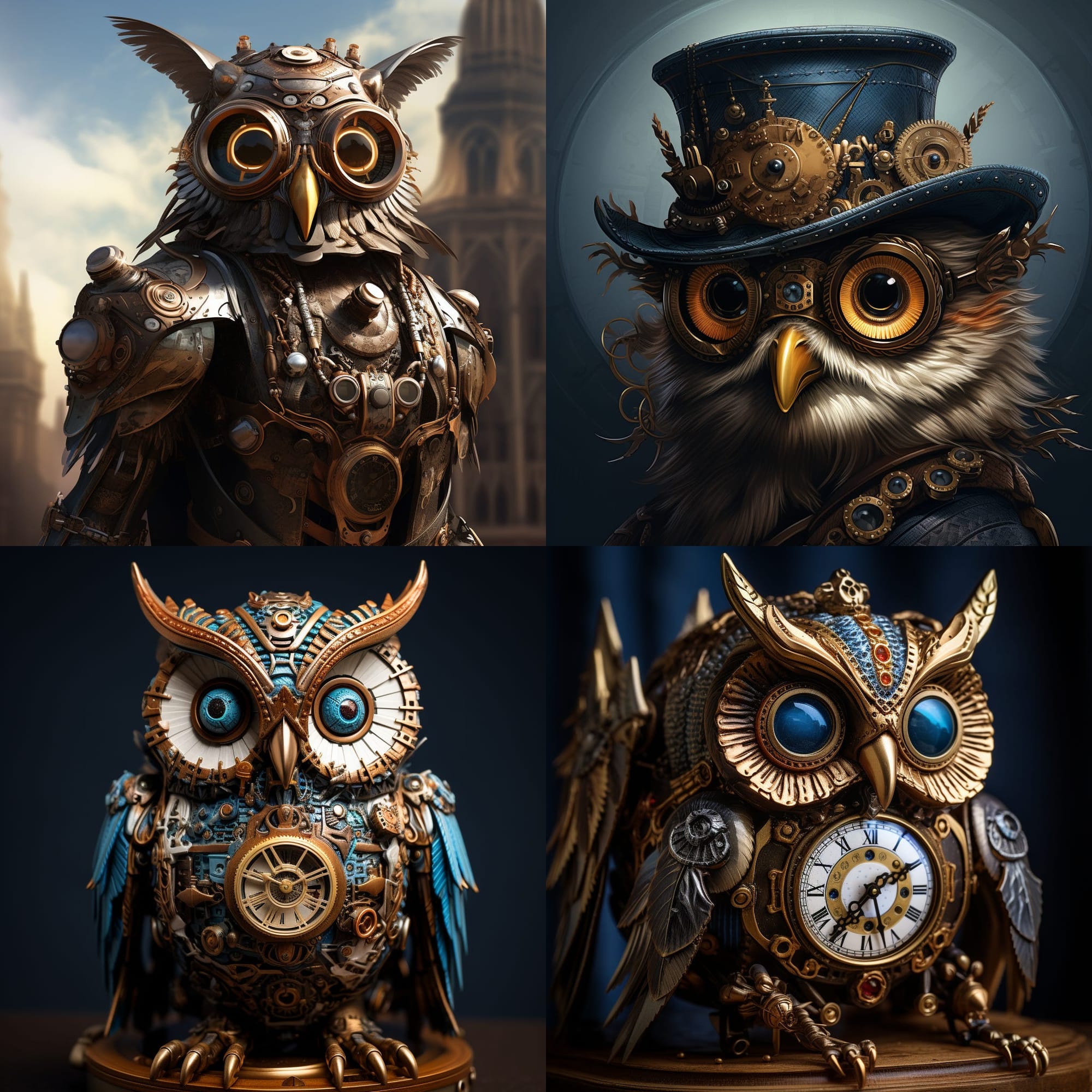
In the space of 12 months, AI systems for generating images and text have gone from interesting and at times exciting, to routinely brilliant. Some of the prose, images, and videos that systems such as Midjourney, DALL-E, ChatGPT, and others now create can seem not only very much something a human would create but also deeply surprising.
A common question is, though, are these systems “creative,” or are they simply “remixing things they have been fed” (leaving aside the fact that this remixing is arguably a form of creativity)? Other people go as far as to say that an AI system could not be genuinely creative because it lacks either an understanding of what is being created or consciousness to be able to appreciate it (and even murkier can of worms!).
On the counterargument side, AI has scored exceptionally well in some early studies of creativity (University of Montana, HU-Berlin, and University of Essex). It’s also hard to argue that there is no creativity in an approach that can create unique packaging for 7 million jars of Nutella.
So, who is right?
The critical first step is to determine the definition of creativity we discuss. As we’ll see, though, even if we take some of the most stringent definitions, it will be increasingly hard to argue AI isn’t creative in the truest sense of the word.
Before we start, a heads up to others who have already written on this topic, including rez0, Ahmed Elgammal, Gunnar de Winter, Keith Kirkpatrick, David Vandegrift (*), and Margaret Boden, as well as the whole field of Computational Creativity which goes far deeper than I do here, hopefully, though, this piece will lay out the main issues in a logical way and arm you with interesting points for the next bar-room or board-room debate on the topic.
(*) David Vandegrift also uses AlphaGo as an example - top marks!
Defining Creativity
This AI creativity question stems partly from pure curiosity, partly from arguments about copyright (if the AI is truly creative, shouldn’t the work be copyrightable?), to our human thoughts about being unique. Creativity feels like a superpower that humans have; if machines now also “have it,” how does that make us feel?
If we were to define creativity as the “creation of ideas” and stop at that, we’re already there.
Digging deeper though, we get more detailed definitions:
Creativity (noun) - Dictionary.com
- the state or quality of being creative.
- the ability to transcend traditional ideas, rules, patterns, relationships, or the like, and to create meaningful new ideas, forms, methods, interpretations, etc.; originality, progressiveness, or imagination.
This gives us a sense that a key part of creativity is “newness versus expectation.” In other words, we are not just talking about something that can be calculated in a straightforward way. it must be something that is not hard-coded into the system or have a straightforward path from query to result.
The definition also highlights something we’ll come back to later: the observer matters since what is traditional for one person may be highly novel for another.
In Solsona (a town in Catalunya, Spain), it is a custom to hoist a papier mashé donkey up a tower every year and have it “pee” down water on the townspeople from the top. This is traditional in Solsona and (trust me) a novel surprise to the visitor. So the observer has in mind a set of rules, traditions, methods, etc., yet they likely don’t capture all possible behaviors of a system. Being outside that norm creates surprise.
From psychology literature (a great starting point can be found here), we generally get a more nuanced take. At the top level, creativity requires two things:
- Newness: the creation of novel or new things (at least to the originator, so-called P-creativity, but ideally to the whole of history H-creativity).
- Value: that the creation be somehow valuable, beautiful, or fit for purpose.
Though there is debate on whether value really has a place, it is generally accepted that it does since, without it even irrelevant ideas would be creative. The definition is often further extended to include the need for the following elements:
- Surprise: something not merely new but not obviously derivable by known rules from something else.
- Originality: that something is not only new to an originator (P-originality) but that it must be assured that it has not been copied verbatim from somewhere else.
- Spontaneity: that the idea created not be, in some sense, a pre-planned answer ready and waiting to be synthesized when a specific question is asked.
- Agency: that the idea be created by a coherent functioning entity and not (for example) by a random natural process. In this sense, one would not call a specific snowflake a creative output. (Though perhaps one would say it is nature’s creation or God’s creation.)
Margaret Boden’s 1998 paper “Creativity and Artificial Intelligence” is also commonly cited and breaks down the idea generation step as coming in three flavors:
- Combinatorial Creativity: results that are novel (even surprising) combinations of familiar ideas. (**)
- Exploratory Creativity: results that are explorations in some structured conceptual space. In other words, something like one concept is applied to another concept in a way that is consistent. The results are novel and can be surprising, but once grasped, they align with accepted thinking styles.
- Transformative Creativity: results that transform one or more dimensions of the space of results to create structures that could not have arisen before. In general parlance, one might characterize this as completely “out of the box.”
In Boden’s paper, she also underlines the importance of creativity, requiring the ability to evaluate whether a given solution is useful or not. Something that is often harder than simply creating answers.
I would argue that spontaneity and Agency are not really at issue here. A lack of spontaneity would imply an algorithmic, pre-planned solution to every prompt. This is clearly not the case. The number of possible prompts is so large that no system designer knows what to expect when many are run, and randomness in the process changes the outcome each time (assuming the LLM temperature is not zero). Agency also seems obvious since a single system has been designed to answer these questions.
Hence, we’re left with a few key considerations to bear in mind:
Idea generation in three ways
Combinatorial
Exploratory
Transformative
Surprise
Originality
Idea evaluation
As a last fun definitional fact, the Oxford Reference dictionary seems to be hedging against machines:
Creativity (noun) - Oxford Reference Dictionary
The production of ideas and objects that are both novel or original and worthwhile or appropriate, that is, useful, attractive, meaningful, or correct. According to some researchers, in order to qualify as creative, a process of production must in addition be heuristic or open-ended rather than algorithmic (having a definite path to a unique solution). See also convergence-divergence, multiple intelligences, transliminality, triarchic theory of intelligence. creative adj.
The clause against algorithmic results is the lay translation of the spontaneity requirement. LLMs are not algorithmic in their internal execution, except in the trivial sense that all computer programs are algorithmic. There is randomness in their paths to an answer under normal operation, so more than one outcome is possible.
Let’s push on and get creative…
(**) I stick with the word idea here since it’s widely used in Computational Creativity, but the result can be something in whatever medium is relevant: text, images, video, sound, etc.
Stunning AI Art
What is obviously true is that AI has already begun to help human artists push the boundaries of what can be achieved. Just like all the tools that came before, stunning new works have become possible. A couple of examples are Refik Anadol’s WDCH dreams and Sougwen Chung’s people and machines works.
WDCH Dreams by Refik Anadol, a 2019 projection on Walt Disney Hall, was constructed by algorithmic processing of the LA Philhamornic’s 100-year archive of recordings. The team took the 45 terabytes data archive and fed this to a neural network to create a learned/interpreted sequence that could be played out on the exterior walls of the concert hall.
By definition, it wouldn’t be possible to do this without neural network technology. However, more importantly, from a practical standpoint, the technology allowed the artists to explore a much wider range of outcomes than they could otherwise.
Sougwen Chung’s 2018 work combines human painting and drawing with a robot arm and mobile robot painting that reacts to her forms.
Both of these sets of works came before the current explosion of LLMs. It’s now routine to see stunning images and 3D work. A couple of my current favorite examples are Hachiman, God of War by user Nerada on Staarri (more of Nerada’s work here), and face/emotion pieces like Embrace by Nightcafe users Sithiis.
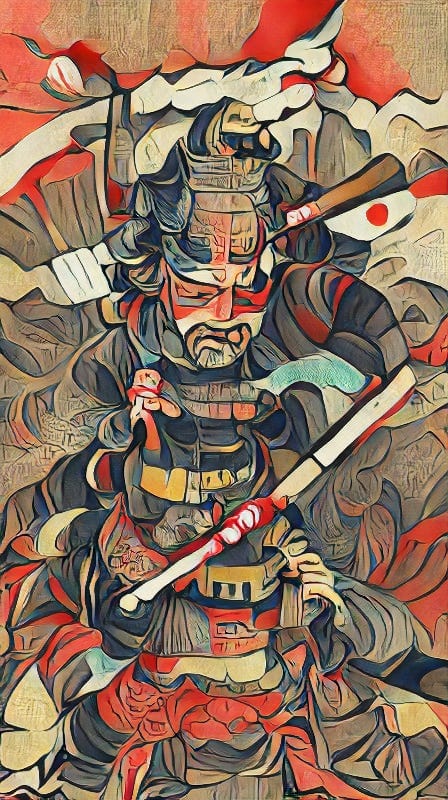
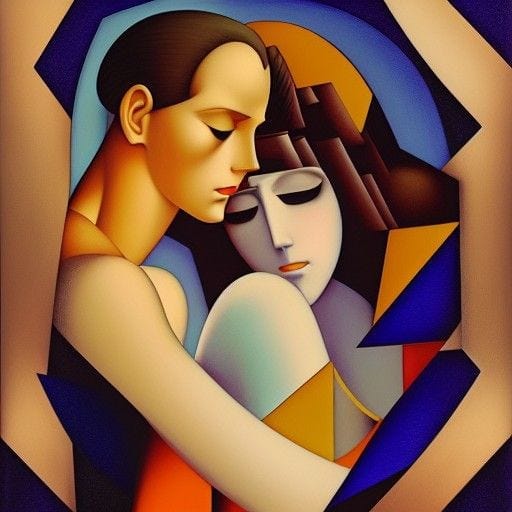
In all of these cases, we’re seeing a system of a human artist working with a machine tool to create an output. With a human in the loop, it’s hard to argue that any of the tenets of creativity are violated.
Fully Autonomous Art
Despite the power of these results, though, these works of art are not “fully autonomous” in that they are the work of an artist using AI as a tool. If we’re trying to evaluate the creativity of AI itself (rather than a human-AI hybrid system), we need to look at the output of such systems when they are not being prompted in complex ways, postedited, or having results cherry-picked.
A few years ago, this would have given risible results. Now, however, the results are often just as stunning as those produced by human+artist combinations.
The following images are from Midjourney using a single prompt (which generates 4 results), no cherry-picking, and no re-rolls.
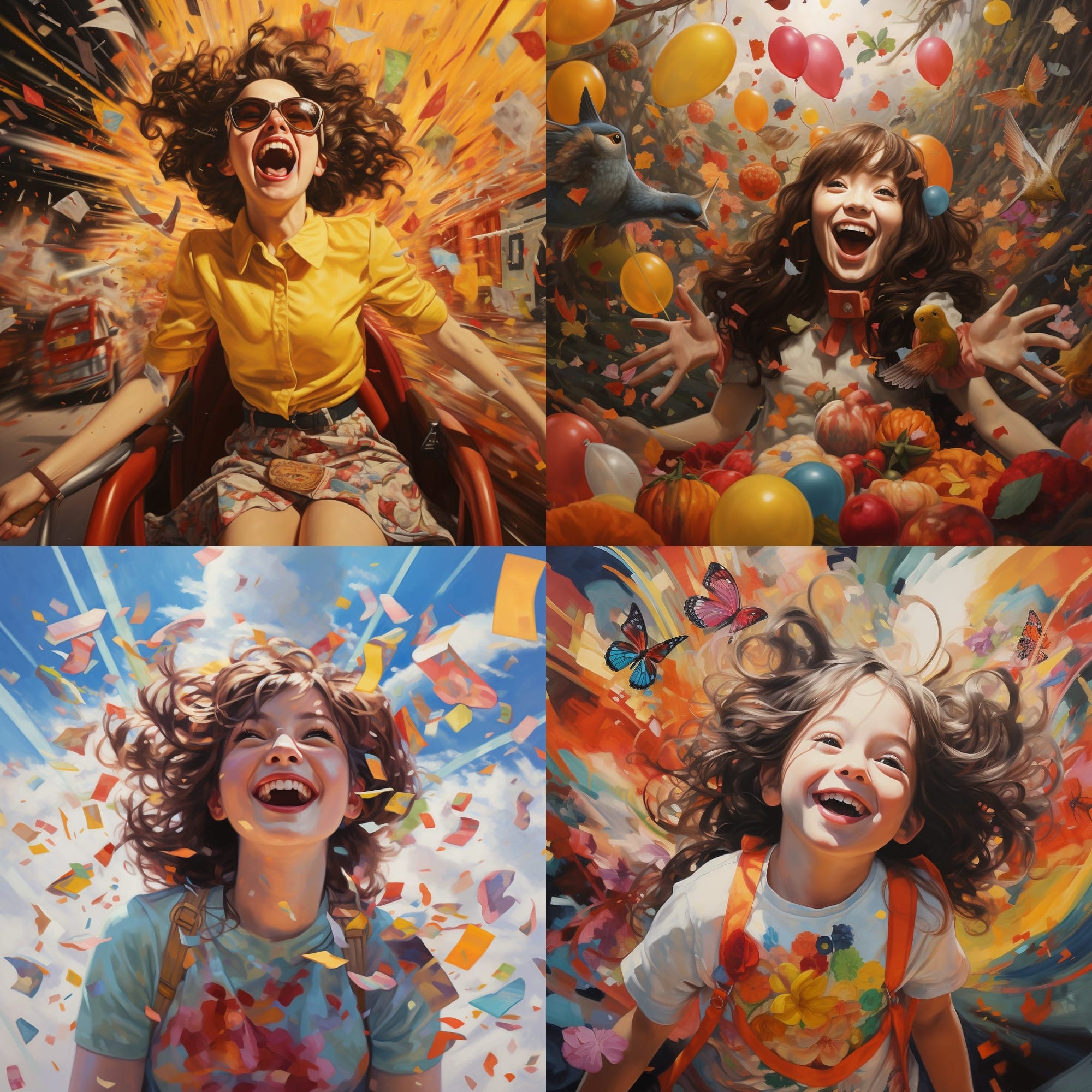
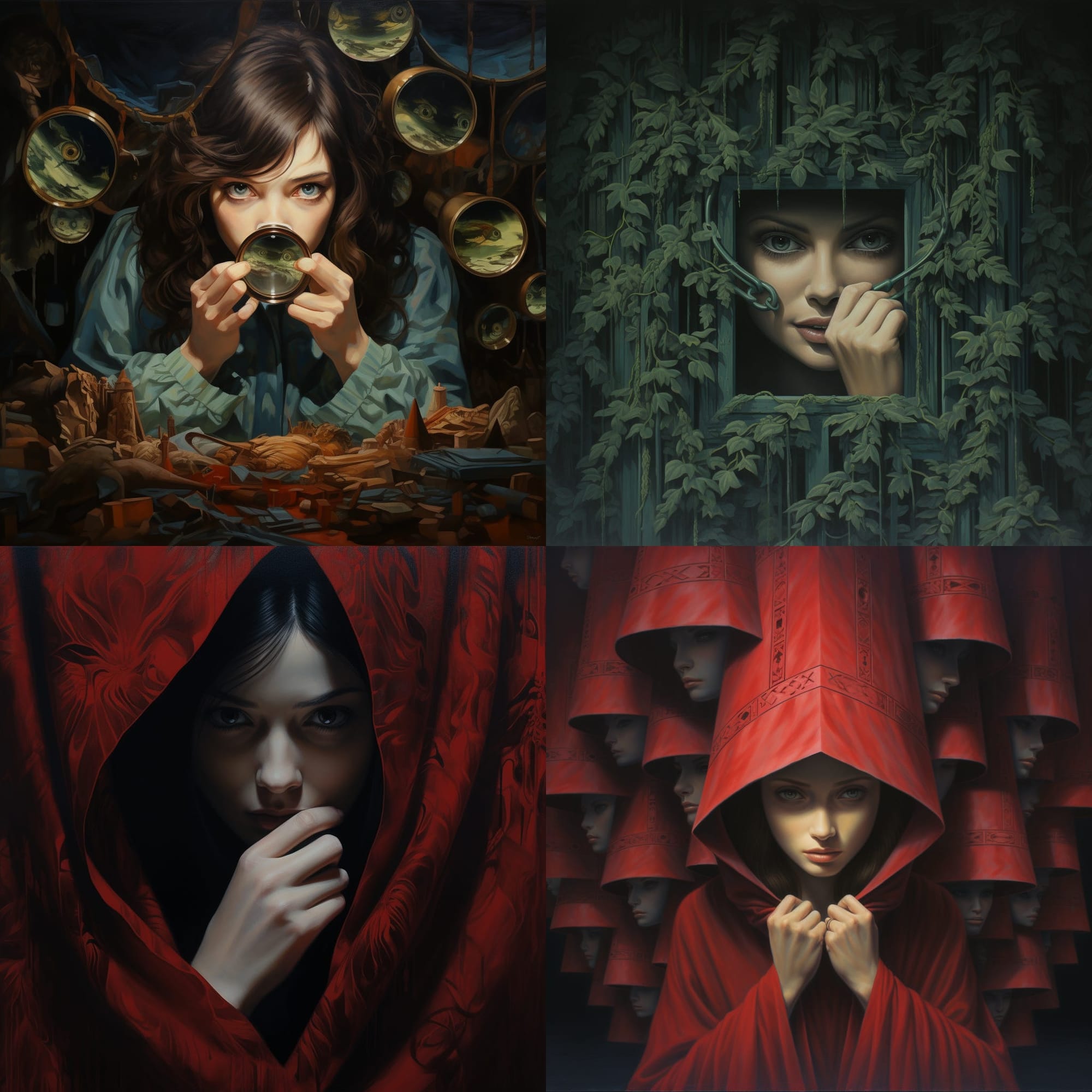
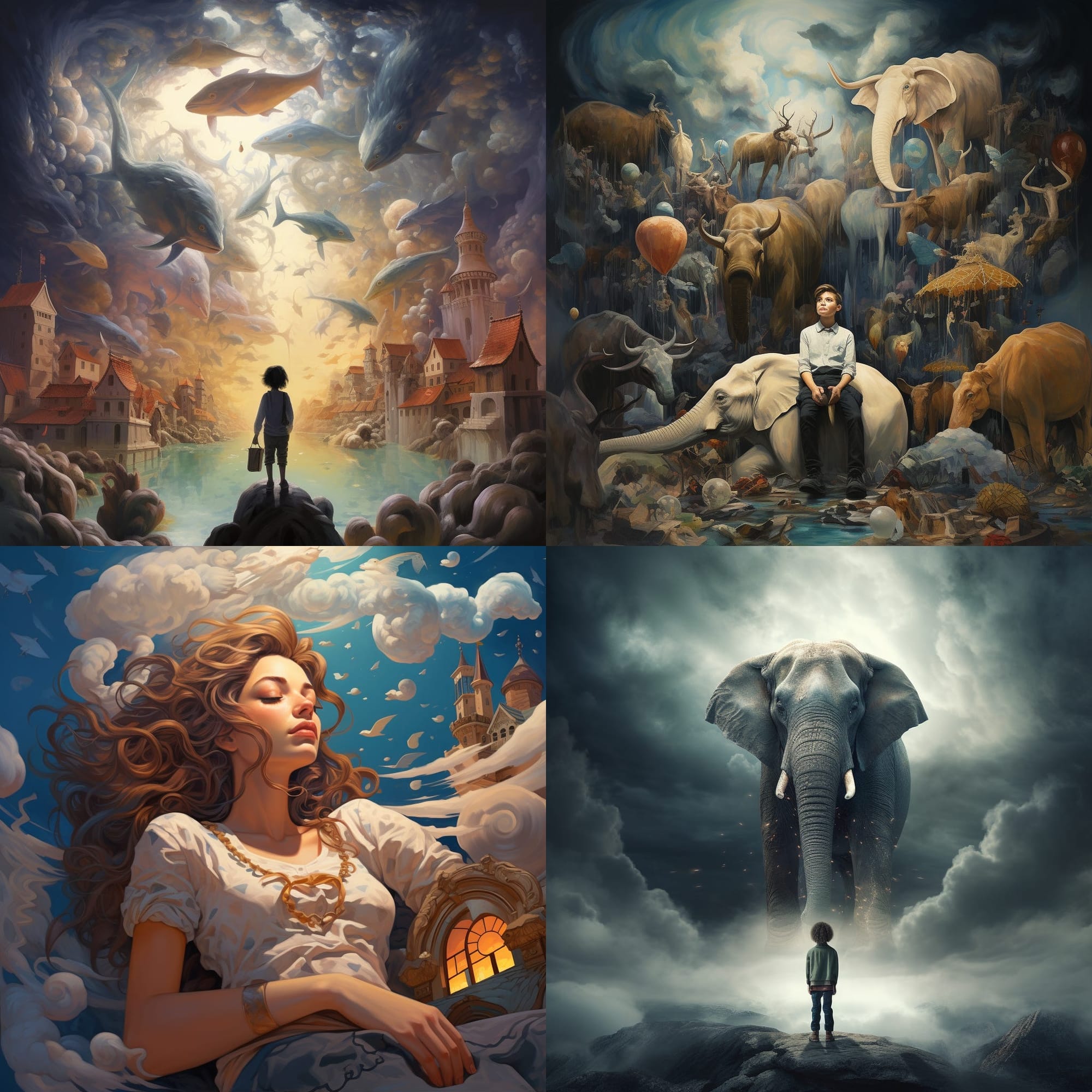
Things are also interesting when prompting using nonsense words for things that do not exist in the real world:
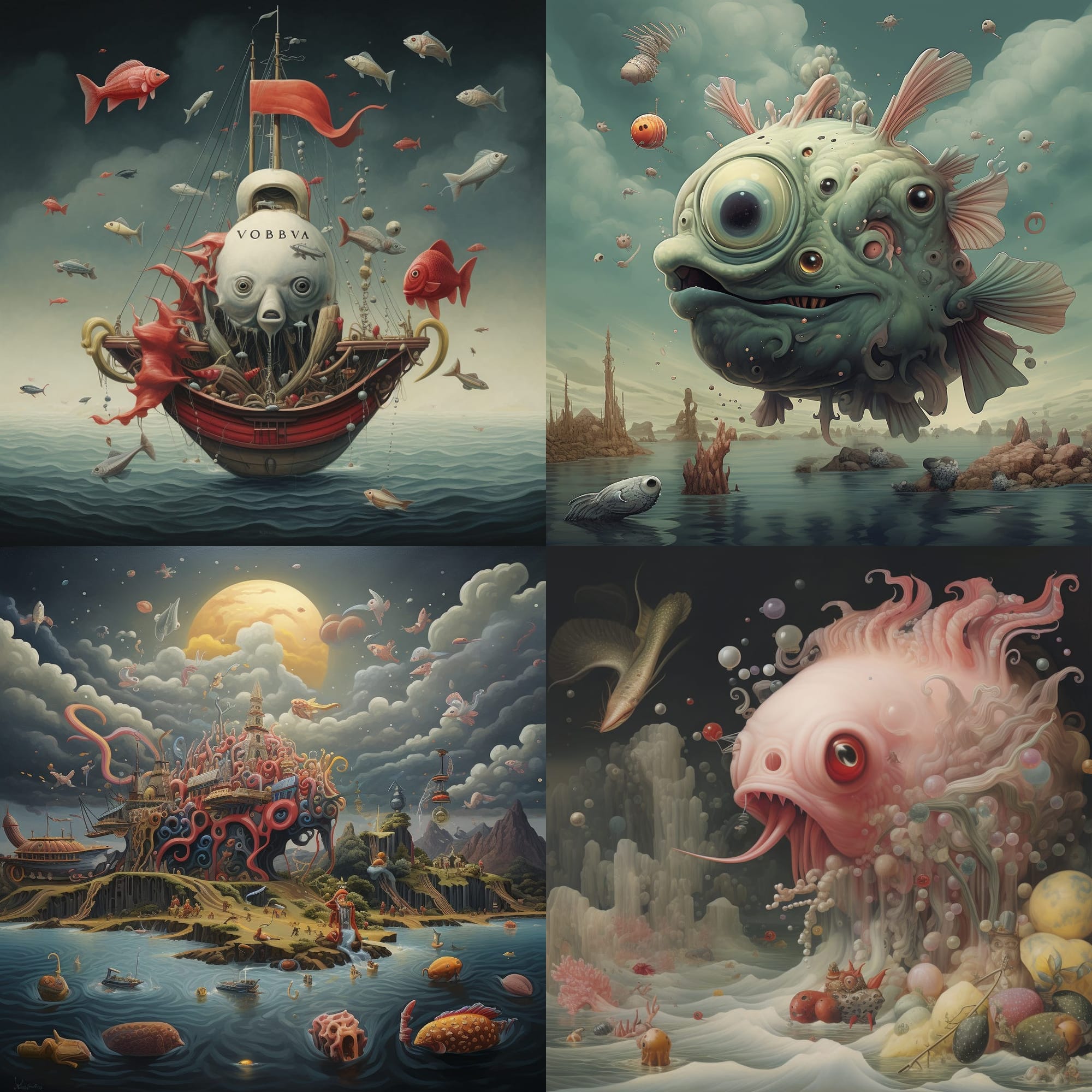
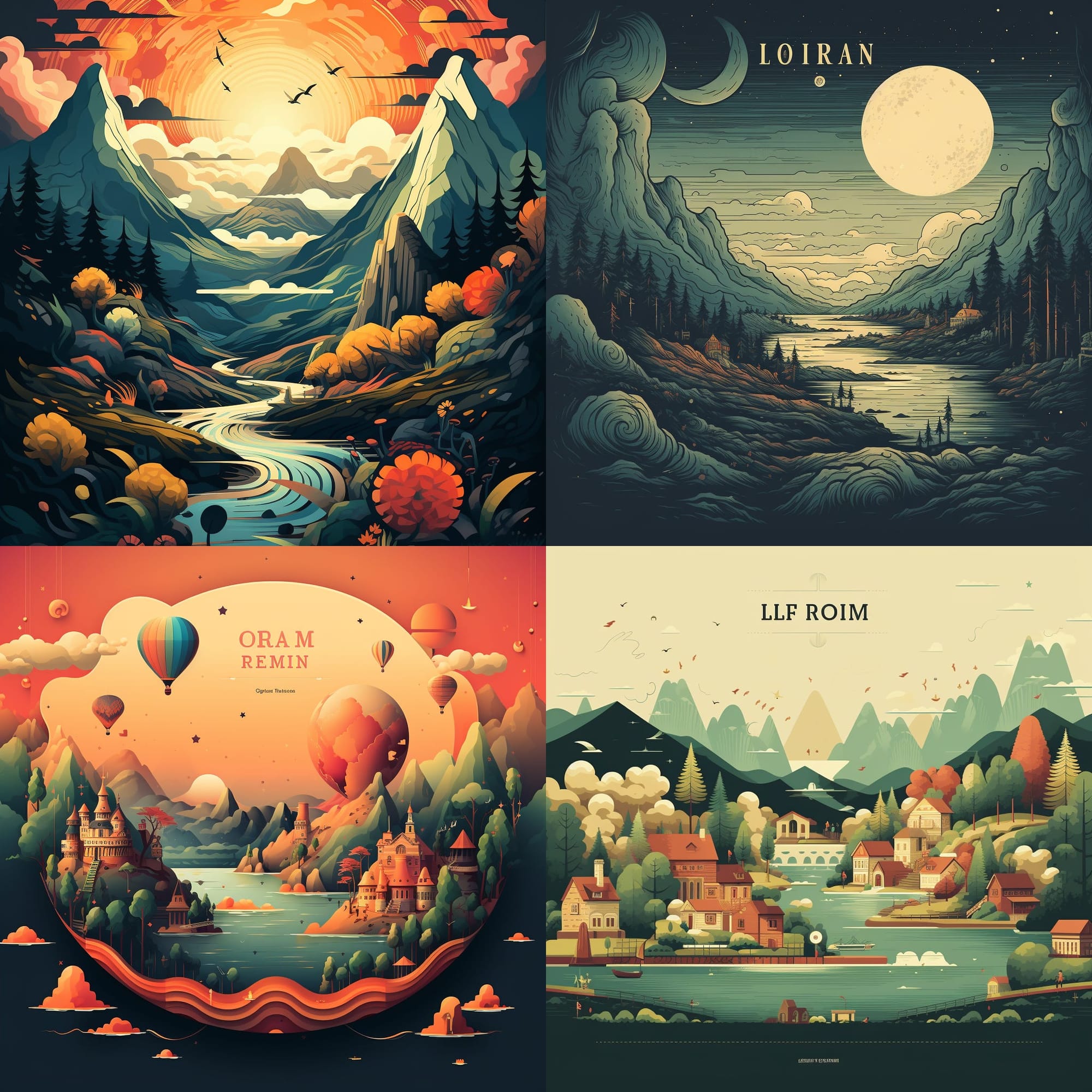
In this simple creation test, it’s hard to say anything specific about why certain prompts result in certain outcomes. Many re-rolls might bring out different results. This only underlines the fact, though, that the system is exploring the space of possible images in a way that is surprising.
Used this way, Midjourney is:
- Exhibiting ideas creation through combination.
- Generating images with recognizable use of concepts (the elephants, children, and use of scale combined in the “Big Dreams” images, for example).
- Tapping into some echoes of meaning even in nonsense prompts. Despite the fact that Yodubabcus Ya doesn’t exist, it seems to resonate more as a creature than a location. Lorem Ipsum is the opposite.
These points don’t only apply to Midjourney but any current generative AI system. Similar phenomena will likely arise.
Inhuman but Beautiful: Move 37
At this point, we might be starting to think that, indeed, AI systems can produce results that will make a human observer say, “Wow, that is novel”. What about the other criteria, however? Being able to evaluate results? Creating not just by remixing but through a significant paradigm shift?
I believe these things are happening in AI Art systems today, but it would be hard to pin them down.
Instead, in this section, we’ll shift to a different domain: the game of Go.
Go is one of humanity’s most ancient games and computationally extremely complex to analyze (many orders of magnitude harder than chess). For many years, creating AI go-playing machines that could get close to beating human Champions was considered out of reach.
Yet from 2010 onwards, Google’s DeepMind team began to make significant progress in using machine learning to train its AlphaGo system over millions of simulated games. Then, in March 2016, the system beat Go Champion Lee Sedol in a best-of-five series.
What’s interesting here is not that Alpha Go won, or even how Alpha Go was built, but how it won. Alpha Go had won the first game with strong and persistent play, but it was in game two that the real shock came. The game was finely balanced, and then, around 1hr into the game (move 37, to be precise), Alpha Go played a move that at first looked like an error.
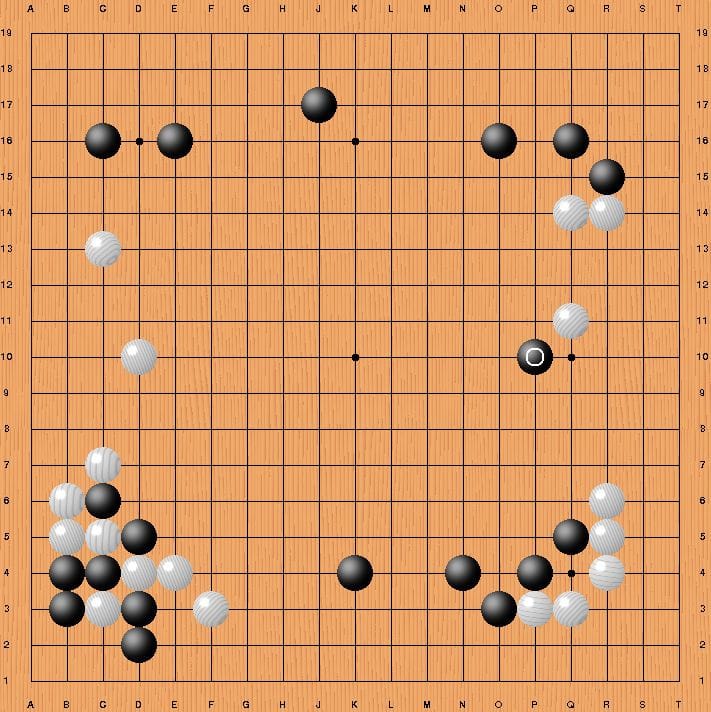
Wired magazine’s commentary:
"That's a very surprising move," said one of the match's English language commentators, who is himself a very talented Go player. Then the other chuckled and said: "I thought it was a mistake." But perhaps no one was more surprised than Lee Sedol, who stood up and left the match room. "He had to go wash his face or something---just to recover," said the first commentator.
Even after Lee Sedol returned to the table, he didn't quite know what to do, spending nearly 15 minutes considering his next play. AlphaGo's move didn't seem to connect with what had come before. In essence, the machine was abandoning a group of stones on the lower half of the board to make a play in a different area. AlphaGo placed its black stone just beneath a single white stone played earlier by Lee Sedol, and though the move may have made sense in another situation, it was completely unexpected in that particular place at that particular time---a surprise all the more remarkable when you consider that people have been playing Go for more than 2,500 years. The commentators couldn't even begin to evaluate the merits of the move.
Yet, over the next three hours, Alpha-Go won the match, and move 37 proved to be a key linchpin in its strategy.
This event may seem extremely esoteric, but it arguably shows clear creativity on the part of the AI:
- The solution was novel and shocking to everyone present, including expert commentators.
- It very likely sprang from millions of games AlphaGo had played in training with itself, none of which had involved humans.
- To be so highly valued as to buck common wisdom and still be a great move suggests AlphaGo’s ability to predict the benefits of the move was extremely strong.
Wired digs deeper, and in talking to the Alpha Go team post-match, the program clearly valued this move highly, knew (via a scoring mechanism) that it was very unlikely a human would make this move (which was an inhibitor to making it), but made the move anyway.
One of the expert commentators that day said: “It's not a human move. I've never seen a human play this move," he says. "So beautiful."
Pulling off such a move in a high-stakes game of Go is impressive, but it would be tempting to say that even if this move is “creative,” Go is a limited domain, and therefore, it’s not clear this ability would generalize to other domains. However, although Go has a very simple set of rules, it is an extremely complex game:
- The number of possible game states is 2.082×10^170 (approximately 2 followed by 170 zeroes), and the number of legal states is around 1% of this (still 168 zeros), larger than the number of atoms in the known universe.
- The number of moves played in a typical game is typically well into the hundreds, and moves played early can absolutely affect moves played later on.
- This creates an extremely complex space of possible actions and outcomes.
The important lesson here is not just that a machine was able to produce a novel, unexpected move and that this move was an extremely strong move at that point of the game. It is also that the machine was able to evaluate the strength of the move and determine it was the right one to make.
Further, the human observers present all understood the power of the move once they had processed it. There’s a strong argument then that Alpha Go’s moves were:
- Novel: It’s extremely unlikely that this specific game position and this specific move had ever been played in any human game of Go.
- Surprising: Alpha Go’s opponent was so shocked he had to consider his next move for a full 15 minutes.
- Original: It’s safe to say no one in the room was expecting this move.
- Spontaneous: Given the vast number of positions of a Go game, Alpha Go does not store all possible board states and the right moves. They are evaluated in the moment. Even after just a few moves in a game, it is extremely unlikely an identical position has been seen before.
- The work of an agent: a single entity making the decision.
- Valuable: The move was clearly powerful.
- Evaluated: AlphaGo had calculated the low likelihood of a human making this move (about 1:10,000 against, according to Wired), but the intrinsic high potential impact of the move pushed it to make the move anyway.
One can also look at Boden’s three types of idea creation and recognize something of them in AlphaGo:
- Combinatorial: Many of the moves during play are combinatorial; this is especially true in Go openings and endgames. Players and AI typically learn patterns that they adapt during play.
- Exploratory: Go has many powerful concepts, such as the notion of “influence” on the board from one stone. These are emergent from Go’s rules and mastery of the game. AlphaGo is highly attuned to these concepts and very likely has pathways within its neural network with the most powerful of these concepts.
- Transformative: This last point may be debatable for AlphaGo since, despite the radical departure of its move from the norm, it still remained within the rules of Go. One might say that the move was as radical as it gets without breaking out of the sandbox of game rules the system must play in to compete.
In a poetic twist, the series also led to another iconic move. This time by Lee Sedol in game 4 of the series (after the series was already lost). This move (move 78 of Game 4) was dubbed “God’s touch.” The move was also brilliant and deviated significantly from the norm. Sedol likely felt unburdened from standard play and played a move that AlphaGo would not expect a human to make. AlphaGo conceded shortly afterward, seeing that its resulting position was lost. Perhaps machines can also help bring out more creativity in humans.
A Vast Search Space Being Explored
Go might seem like an abstract world to go into when we started with pictures of balloons and butterflies. But, there was a method in the madness.
The Go example underlines a fundamental point in understanding the structure of creativity. An idea (or image, song, video, etc.) is the answer to a question drawn from a space of all possible answers.
This space can be thought of as an n-dimensional matrix of all possible responses. One way to interpret n is the size of the output vector. In Go, this is a two-dimensional output as a coordinate on the Go board. I.e., “place my next stone at (3,5)”. For a Midjourney image, it is the vector of the color value (itself a vector) at every pixel on the output image. There is also another interpretation of the n-dimensional space: as the vast matrix of all the concepts, partial solutions, and other artifacts along the axes and all of their possible combinations of those elements.
Either way, searching for an idea to solve a problem involves searching such a space.
For almost any problem, the number of answers to the question is potentially vast. In theory, any point in N-dimensional space could be an answer, though an extremely high percentage (99.999% and more) will be very poor answers.
This is the crux of understanding creativity.
When tasked with the question “Visualize an owl,” the problem can be approached in different ways:
- A non-artist may explore a space that is relatively close to the common notion of an owl. They might call up pictures of natural owls, may sketch likenesses, and ultimately return something satisfactory. The result would likely match the question but not be considered particularly creative.
- A trained artist would likely explore the space in a way that goes significantly beyond what the non-artist may do. Drawing on their unique style and experiences, as well as performing a wider search of the possible space of owls. The result will, in many cases, be thought of as creative and beautiful.
- An AI system is likely to explore again different portions of the space of possible owls. Drawing on fragments of previously learned pathways, the resulting owls will likely look different to human-created results. Evaluation functions will likely choose some that are at least close to answering the challenge.
Thought of this way, it seems evident that what generative AI systems give us is an idea-creation machine that explores the space of answers in a way that can go well beyond what a human would do by themselves or at least be very different.
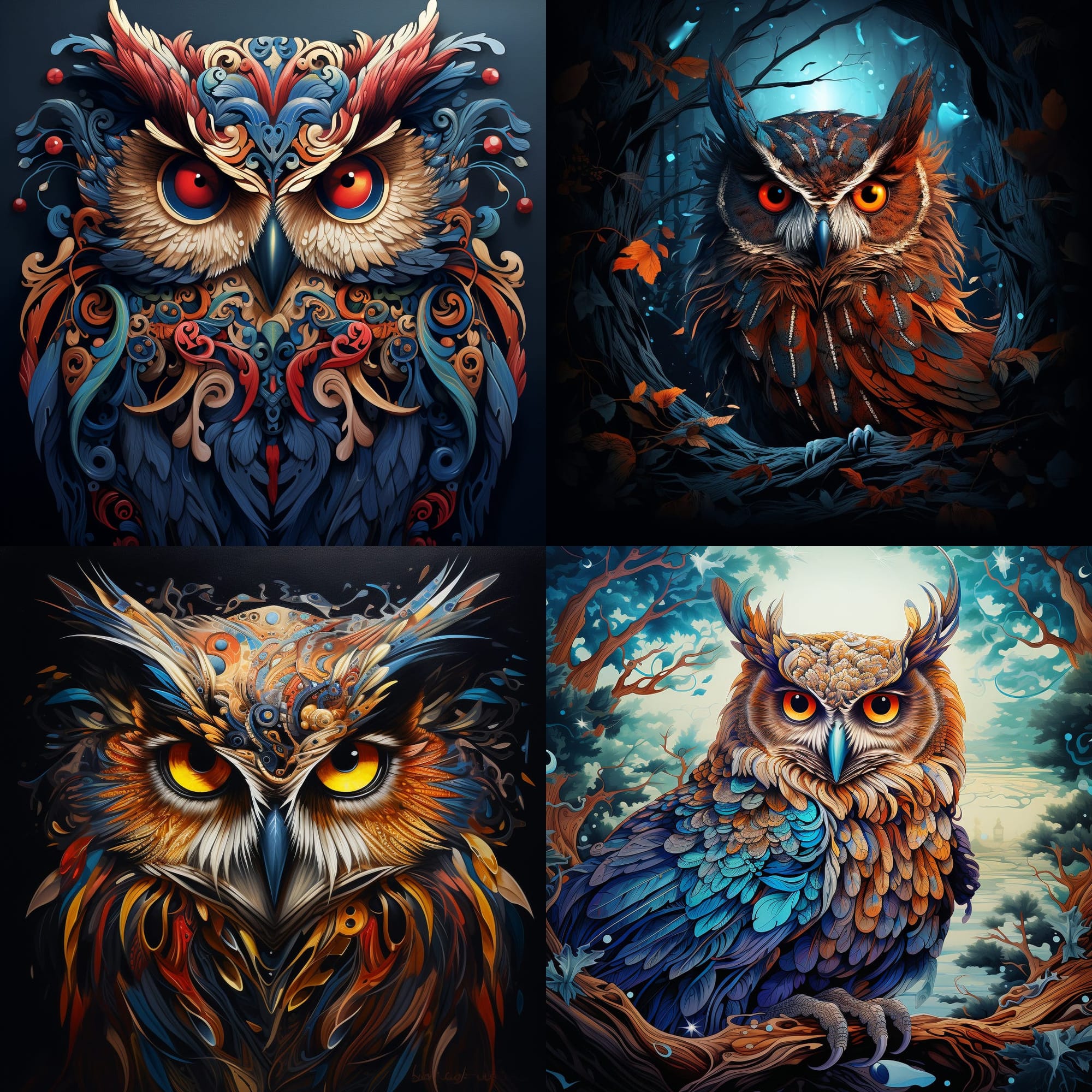
Many of the results created by the machine may look strange or perhaps deformed, but some will hit the mark.
These owls clearly seem to pass the tests of:
- Novel: No one has seen these owls before.
- Surprising: Given any group of non-artists and artists, it’s likely that some of the created owls will be from parts of the solution space they never even considered.
- Original: They will not simply be retrievals of whole images from the training data (LLMs do not store the training data or act as a database).
- Spontaneous: The LLM has no hardcoded pathway within it to create any of these specific images; they are emergent based on the prompt.
- The work of an agent: The LLM is clearly acting independently and as a closed system.
- Value: Assuming quality similar to today’s image generators, chatGPT, and other systems, it’s highly likely some of the images will be high enough quality for people to wish to use them in prints or displays. (See Midjourney’s Owls above.)
- Evaluated: AI art generators often still produce failed images. However, even these are in some perceptible way “close” to the challenge set (the prompt). The LLM’s entire structure is an evaluation mechanism to try to find something that is as close as possible to an answer to this prompt as it can.
In terms of Boden’s three evaluation types, there are clearly combinatorial and exploratory types of creativity going on (see the “Big Dreams” example above again).
The transformative level is admittedly more subjective in this case. However, these types of extremely surprising transformations do happen. My favorite examples of the moment are harvest meme images:
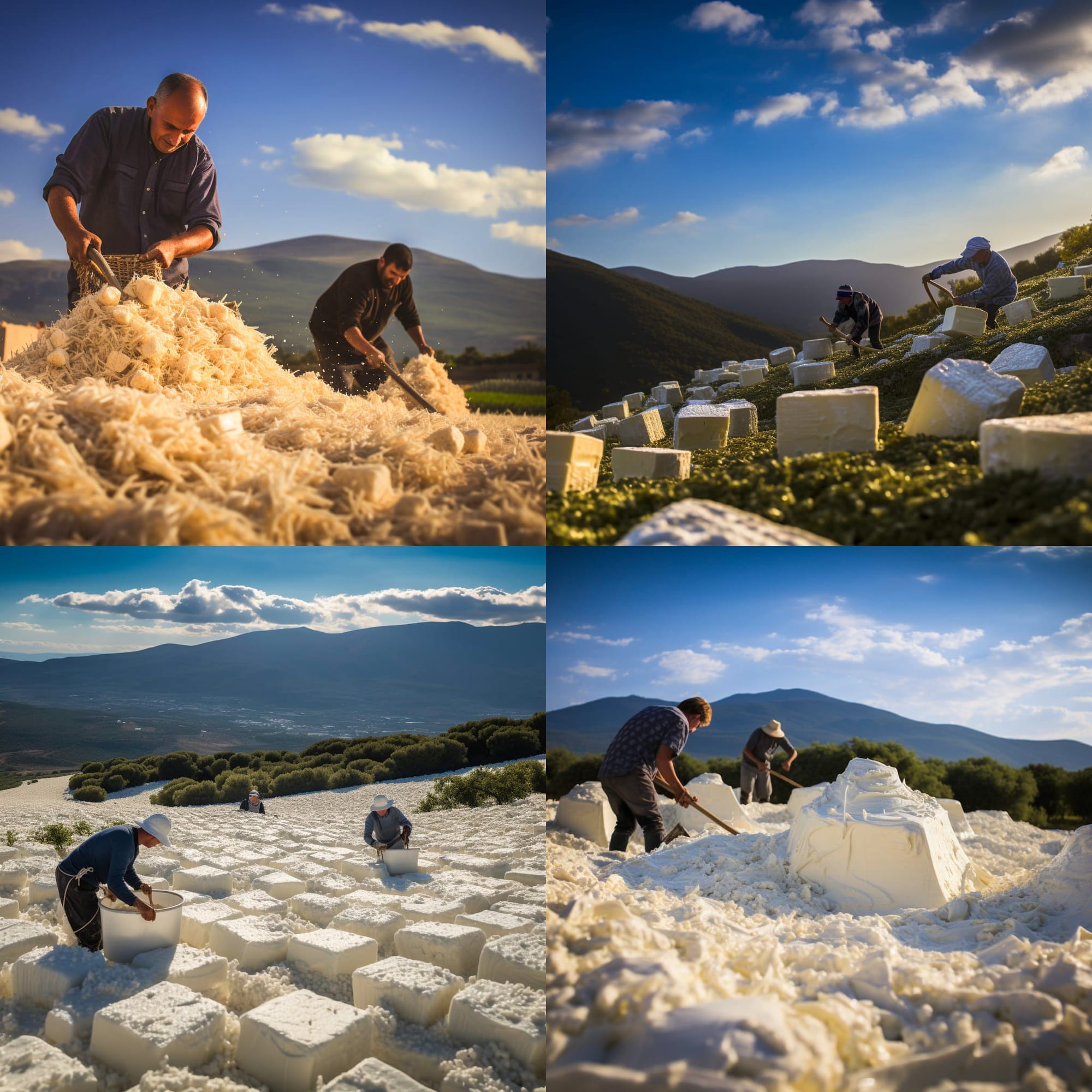
In cases like these, the images being generated clearly violate some obvious rules about the world we take for granted. For some applications, this will make them useless or even dangerous (explaining Greek culture, for example), but for others (humor in this case), it’s spot on and extremely surprising.
Counterarguments
Even though today’s LLMs align broadly with the standard definitions of creativity, there are still more counter-arguments. The most common these are:
- “The system isn’t truly autonomous; it needs a prompt as input, and half the artistry resides in that.” Some prompts can indeed be highly creative and steer an AI system. However, it’s also clear that today’s generators will generate things even if prompted with nonsense (see the two examples above). Artists often start a project with something in mind (the Sistine Chapel was commissioned, not painted on a whim). Lastly, if spontaneity in prompts is really required, it would be trivial to rig up a prompting bot that randomly takes the most common phrase on major news channels every hour and uses this as a prompt. (Or perhaps for more calming results, the gardening channel.)
- “The system has no understanding of the concepts involved, so at best, it’s carrying out some kind of mimicry which is not creative.” This is a more profound claim. LLMs do, however, have some fuzzy model of the world (see my previous lengthy post here). The model is derived from vast numbers of correlations between images and text. Whilst these systems perform quite poorly at causal reasoning (and maybe always will in their current form), they are still clearly implicit truths about how concepts relate embedded in the network. This means that many of the images make logical applications of concepts to one another.
- “The system produces a lot of poor answers. It clearly can’t evaluate which answers are good.” ChatGPT already catches itself and uses a multi-model architecture for specialist tasks, so there is self-censorship going on. More importantly, the whole structure of an LLM is designed to give answers that are “close to” the output humans might expect. In some sense, the whole network is designed to evaluate itself at every step. It may still often fail, but as the technology develops, the hit rate will likely continue to improve.
- “The system isn’t conscious, so any art it produces won’t have the element of soul that a human-created piece does.” This is a hard one to refute since it defacto links creativity to consciousness, which is a whole other lengthy debate. It may mean, for example, we’d need to determine if crows are conscious since they can work out how to creatively use tools, as well as a myriad of other such questions.
Beauty in the Eye of the Beholder
The last meaningful question seems to be around the notion we get from the dictionary definition of creativity.
Are these systems really fulfilling the novelty definition as described here?
the ability to transcend traditional ideas, rules, patterns, relationships, or the like, and to create meaningful new ideas, forms, methods, interpretations, etc.; originality, progressiveness, or imagination
It seems clear to me that this is, in fact, a relative question and depends heavily on the eye of the beholder.
- In a constrained domain such as Go, it seems only fair to acknowledge when a system has broken out of the generally accepted frameworks of understanding the game. AlphaGo clearly did this.
- In more open domains such as text generation, image generation, and others, the question is what is “expected” and “traditional.” This changes for whomever is writing the prompt.
In the late 1990s, when Margaret Boden’s paper was written, most AI systems were limited in the domain they could operate in. Today, ChatGPT is trained on a vast swathe of human knowledge, and image generators can generate almost any image imaginable.
The goalposts have moved. Now, generative AI systems have horizons that encompass much of human experience, and we, as individual humans, have only ever experienced a limited part of that overall human experience. It is, therefore, completely possible that in many interactions, a human observer will see results that are part of possible experience but which they themselves have never seen.
Depending on the prompts, many things will feel new, surprising, and original.
Why it Matters
As a human being, it’s hard to swallow the idea that a machine with some software based on a snapshot of knowledge could be “creative.” This notion somehow challenges our own humanity. Wasn’t “creativity” the last bastion of what humanity had left that machines couldn’t achieve?
Yet, if you break it down, it’s hard to argue that AI can’t be creative in almost every way we care to define.
This doesn’t mean that our humanity is cheapened, however: We created AI, after all, and for a very long time, the combination of Human+Machine will very likely outshine pure machine results. We have to be a little less superior in our thoughts.
If we retreat to the last bastion of the hold-out position and say that AI cannot be creative because it lacks consciousness, we might feel ok for a while. However, it seems more than likely that saying this will lead us down a path where we’ll have to decide on a definition of consciousness that works both for machines and for us or for neither.
It’s also very understandable that the idea of machines being creative can feel extremely threatening or demoralizing to someone whose livelihood is in an artistic domain. There will no doubt be a great deal of disruption. This creativity, though, doesn’t only apply to inherently artistic fields. It will perhaps be even more important in Engineering, Biology, Ecology, drug discovery, finance, space travel, teaching, and many other areas.
It is exciting to think that humanity has created engines of creativity that are powerful enough to surprise us. In many important fields, it means we’ll be able to explore the space of possible solutions in a much more effective way than before. AI-powered drug discovery, ecological modeling, and engineering are already happening. LLMs will only accelerate that more and put these abilities into the hands of more people.
This turned out to be a long one! Thank you for sticking it out until the end!